About DualSeqDB
DualSeqDB is a manually curated database that contains data of gene expression changes in different bacterial infection models, measured by dual RNA-Seq. It comprises more than 250,000 entries, with information about bacterial and host gene expression levels under in vivo or in vitro conditions. The entries were produced by collecting raw sequencing data from 7 different studies where dual RNA-Sequencing was performed, and subsequently analyzing these data through a standardized pipeline. It includes information on 6 different strains of pathogenic bacteria and a variety of cell types and tissues in Homo sapiens, Mus musculus and Macaca fascicularis at different time-points.
What is the relevance of dual RNA-Sequencing in bacterial infection processes?
The use of high-throughput sequencing (RNA-Sequencing) has unveiled new levels of complexity in the transcriptomic response of pathogens and hosts during infection. Dual RNA-seq has become a leading approach to uncover the intricate relationship between pathogen and host interactions. The term “dual RNA-seq” refers to the process of simultaneously analyzing RNA-seq data of a pathogenic bacteria and the infected host. During infection, pathogens cause a deep transcriptomic remodeling of the host. In its turn, pathogens trigger the expression of unique genes that ensure their survival and allow replicating within the host. In this context, dual RNA-seq allowed researchers to identify “molecular phenotypes” in infection that would remain otherwise undetected.
Background
In a dual RNA-Seq experiment, animals are inoculated with a defined load of bacteria (in vivo) or relevant cell culture models incubated with bacteria at a defined multiplicity of infection (MOI, in vitro). After infection, samples are taken over time to determine the time response. Infected cells are lysed, RNA is isolated and cDNA library is prepared and sequenced using high-throughput sequencing technologies, which generates large amounts of data. RNA-seq of mock-infected host cells and initial bacterial cultures are used as control conditions for expression analysis. Several technical issues need to be addressed in dual RNA-seq experiments, including the different nature and content of RNA between bacteria and eukaryotic cells, the larger proportion of RNA from eukaryotic cells, and the need to account for the prevalence of rRNA transcripts and variable infection rates. Usually, such drawbacks are solved using high depth sequencing, pathogen and host rRNA depletion and enrichment of samples for infected host cells by fluorescence-activated cell sorting (FACS).
The availability of increasing raw sequencing data produced from dual RNA-Seq experiments has motivated the creation of DualSeqDB, a user-friendly platform that allows to search for changes in gene expression during infection at both pathogen and host level. To build this database we analyzed raw sequencing data from heterogeneous dual RNA-Seq studies using a well-defined pipeline, to generate comparable gene expression data. Also, DualSeqDB includes measurements for all genes detected in the experiments, as opposed to the usual summaries published, which tend to include only those genes considered differentially expressed attending to different criteria.
Obtaining differential expression values from dual RNA-Sequencing
To build DualSeqDB, we selected only dual RNA-Seq studies with raw data available, containing a minimum number of biological replicates and where data were available for infected and control conditions of both the pathogen and host. For each study, genome and annotation files were downloaded for pathogen and host from the NCBI Reference Sequence Database (RefSeq). Bacterial and eukaryoric genome indices were created with Bowtie2 and HISAT2, respectively. HISAT2 can take into account alternative splicing of genes and was used for eukaryotic genome indexing. For each biological replicate, raw sequencing reads in FastQ format were trimmed with Trimmomatic to remove adapter content. Afterwards, surviving reads were mapped to host genome index with HISAT2. Mapped reads were stored as BAM files, and unmapped reads were kept in a separate FastQ file. FeatureCounts, together with the host annotation file, was used for gene counting, and a matrix of read counts was generated were each row represents an annotated gene and each column represents a different condition or biological replicate. Unmapped reads from the previous mapping step were then mapped back to the bacterial genome index with Bowtie2, and a matrix of read counts was produced similarly by using the bacterial annotation file and FeatureCounts. Finally, differential expression analysis was performed against control conditions separately for the bacterial and the host matrices by using the DESeq2 R package. A gene expression change value (measured in log2 fold change) and its associated p-value were then generated for each annotated gene with detected reads in at least one condition. Additional information such as bacterial ID, host ID, time-point, experimental condition (in vivo/in vitro), cell type/tissue, etc. was added to each gene to create the final format of each entry in DualSeqDB.
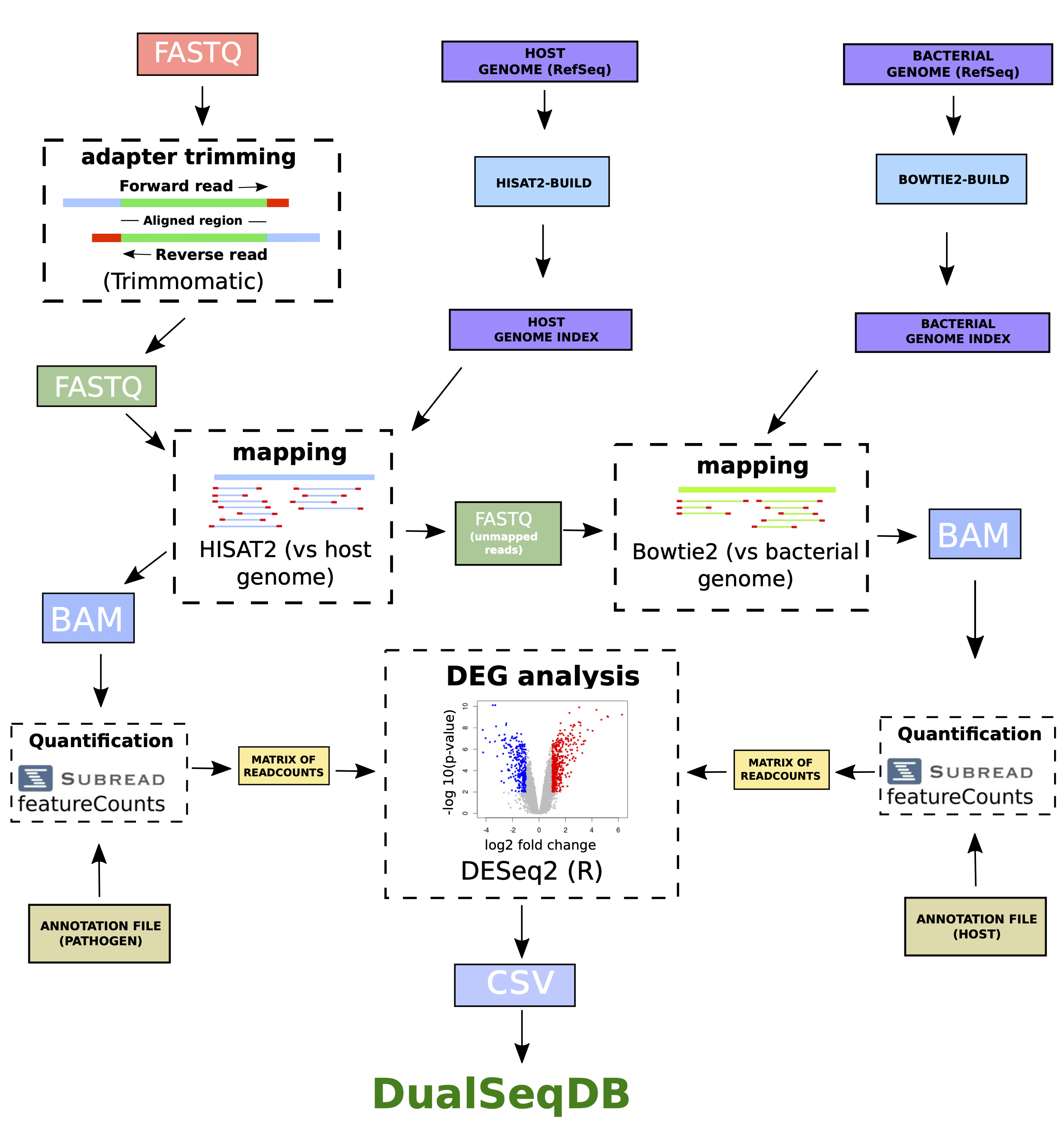
Updates
You can follow us on Twitter at @tartaglialab and @sysbiogr for updates.Submitting data
If you have a dual RNA-Seq dataset you would like to submit or recommend for DualSeqDB, please go to: Submit data. Thank you!Contact
Please feel free to email Javier Macho (javier.macho@uab.cat), Benjamin Lang (benjamin.lang@crg.eu), Gian Gaetano Tartaglia (gian@tartaglialab.com), and Marc Torrent (marc.torrent@uab.cat) — any questions, ideas and feedback are very welcome.How to cite DualSeqDB
Please reference Macho Rendón, J., Lang, B., Ramos Llorens, M., Tartaglia, G.G., and Torrent Burgas, M. (2021). DualSeqDB: a database to assess the relevance of bacterial genes during host infection. Nucleic Acids Res. 49, D687–D693.Primary data sources
- 1. Kachroo P, et al. (2020). New pathogenesis mechanisms and translational leads identified by multidimensional analysis of necrotizing myositis in primates. mBio. 11(1):e03363-19
- 2. Westermann, A. J. et al. (2016). Dual RNA-seq unveils noncoding RNA functions in host-pathogen interactions. Nature 529, 496–501.
- 3. Leon N. Schulte, et al. (2020). An Advanced Human Intestinal Coculture Model Reveals Compartmentalized Host and Pathogen Strategies during Salmonella Infection. Mbio. 03348-19.
- 4. Rienk A Rienskma, et at. (2015). Comprehensive insights into transcriptional adaptation of intracellular mycobacteria by microbe-enriched dual RNA sequencing. BMC Genomics 16:34.
- 5. Nuss AM, et al. (2017). Tissue dual RNA-seq allows fast discovery of infection-specific functions and riboregulators shaping host–pathogen transcriptomes. Proc Natl Acad Sci, 114.
- 6. Damron, FH, et at. (2016). Dual-seq transcriptomics reveals the battle for iron during Pseudomonas aeruginosa acute murine pneumonia. Sci Rep 2016;6:39172.
- 7. Griesenauer, B, et al. (2019). Determination of an Interaction Network between an Extracellular Bacterial Pathogen and the Human Host. Mbio 10, e01193–e01119.
- 8. Aprianto, R, et al. (2016). Time-resolved dual RNA-seq reveals extensive rewiring of lung epithelial and pneumococcal transcriptomes during early infection. Genome Biol. 17(1):198.
Funding
This study has been funded by the Spanish Ministerio de Ciencia, Innovación y Universidades (SAF2015-72518-EXP, SAF2017-82158-R and RYC-2012-09999) and a Research Grant 2016 by the European Society of Clinical Microbiology and Infectious Diseases (ESCMID).
Licence
Our own work is licenced under a Creative Commons Attribution-NonCommercial-ShareAlike 4.0 International Licence
Acknowledgements
For UniProt proteins, a protein visualisation is automatically generated by ProViz from the Davey lab. ProViz is an interactive exploration tool for investigating the structural, functional and evolutionary features of proteins.NCBI BLAST version 2.9.0+ (March 2019) is used to search by sequence similarity.
Template and CSS from Bootstrap, various small icons from Font Awesome and 'Genetic Manipulation' modified from Anthony Ledoux from the Noun Project, table export to CSV files via ExcellentExport by Jordi Burgos, and table sorting via bootstrap-sortable by Matúš Brliť.
See also: the CRG's legal notice. © 2025 tartaglialab.com